The Dynamic high-Resolution dEmand-sidE Management (DREEM) model is a fully-integrated energy demand and demand-side management simulation model, focusing on the building sector, which expands the computational capabilities of existing Building Energy System (BES) and demand-side models, by not only calculating energy demand, but by also assessing the benefits and limitations of demand flexibility, primarily for the main end-users (consumers/citizens), and, then for other energy system actors involved (e.g., suppliers, retailers, DSOs, TSOs, etc.).
Key Features
The main premise behind the development and the use of the DREEM model has been that, in order for end-users to have a more active participation to the energy transition, they first need to become more aware of the benefits from investing in new energy products and services.
In this context, the novelty of the model lies in its potential to be used in a wide range of applications, not only to assess the existing technological infrastructure, but also to support the development of business models and regulatory innovations, which maximise the value of energy products and services, and monetise them, to fairly compensate end-users and other energy market actors.
Overall, the DREEM model:
- Embodies key features towards the simulation of renewable energy, energy efficiency, and other demand-flexibility actions, like demand response, in the building sector.
- Builds on the concept of modularity consisting of multiple components, each of which is composed of additional modules, allowing for more flexibility in terms of possible system configurations and computational efficiency (high time resolution and quick simulations) towards a wide range of scenarios, to study different aspects of end-use and energy transition (Figure 1).
- Provides the ability to incorporate future technological breakthroughs in a detailed manner, such as the inclusion of heat pumps, or electric vehicles, in view of energy transitions envisioning the full electrification of the heating and transport sectors.
- Produces outputs for a group of buildings, for example a neighborhood, a district, a municipality, or an energy community.
- Serves as a basis for modelling domestic energy demand within the broader field of local, regional, and national energy systems in different geographical/climate and socioeconomic contexts. Currently the model’s spatial resolution is mainly limited to European Union (EU)’s Member States, but given the availability of historical data/observations, the model can be expanded beyond the EU.
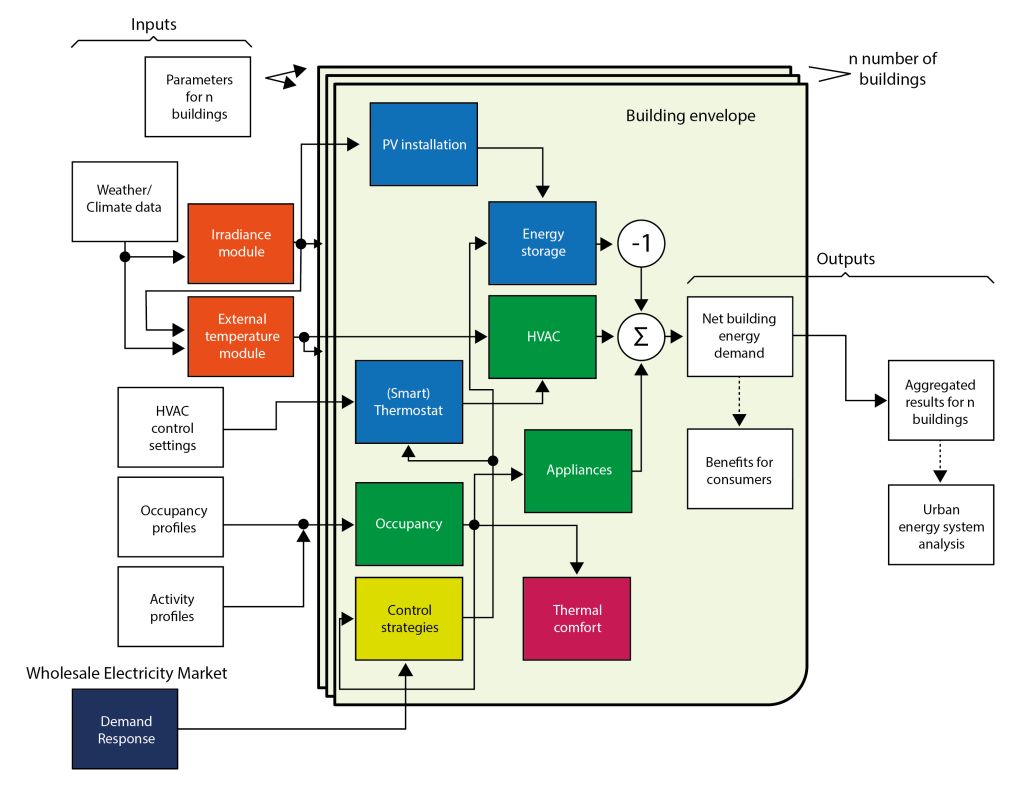
All the modules of the model are developed using the “Buildings” library, which is an open-source, freely available Modelica library for building energy and control systems. Alongside to the Modelica models, Python scripts have been developed to model parts of the model’s components, and to enable the interface with the Dymola simulation environment.
Types of Indicative Applications
Energy Demand Calculation Towards 2030 & 2050
The DREEM model uses building composition and occupancy patterns derived from historical and statistical data, or measurements, based on the concept of fixed, a priori schedules, to produce energy consumption patterns on a selected time step (e.g., 10’, 30’, 60’, etc.) and for a pre-determined time horizon (e.g., 1 day, 1 week, 1 month, 1 year, by 2030, by 2050, etc). Schedules are defined independently of the predicted conditions during simulation, and they represent simplified and predictable activity scenarios according to day types. Schedules derive either from standards or from observation-based statistically aggregated data and include rules, where actions are perceived as direct consequences of one or more drivers. Statistical data is also used to create “activity profiles”, which show people’s tendencies. For example, people tend to do cooking activities around mealtimes, etc. Thus, each activity has its own daily profile. Furthermore, a weighted stochastic function is applied for some days, or hours of the day to make occupants more stochastic in terms of their activity profiles (e.g., after-work/school activities, etc.). Next these activities are linked to appliances and other energy carriers, e.g., heating, cooling, etc.
Prosuming and Energy Efficiency
DREEM simulates small-scale/rooftop PV installations with orientation, taking as input the direct and diffused solar radiation, based on historical irradiance data for the geography of interest, and the location and the orientation of the PV panel. It can also estimate the energy-saving potential of different energy efficiency measures and practices in different localities.
Demand Response
DREEM couples all the important aspects of end-use with a demand-response modelling framework, that builds on the concept of time-based methods; time-based methods are typically considered the most effective demand-side management strategies, as their inherent characteristics are more suitable to real-world unsteady and fluctuating energy consumption patterns. In this context, the model simulates demand-response mechanisms that derived through: (i). considering Hourly Electricity Prices (HEPs) and a Limiting Price (LP), and (ii). a more “real-world” situation, in which a central planner that attempts to maximise flexibility value by issuing demand-response signals, is assumed. This entity learns the optimal policy that maximises its revenues through an optimisation approach based on Reinforcement Learning theory. This is another novel aspect of the DREEM model, since it not only brings together all the guiding principles of demand-side modelling, but it also uses Machine Learning to illustrate the decision-making framework and solve the dynamic pricing problem in a hierarchical electricity market that considers both service providers’ profit opportunities and consumers’ costs.
Energy Management Systems and Consumption Pattern Changes
DREEM can model different energy management supervision/control strategies (algorithms) towards the achievement of energy savings and cost-effectiveness, e.g., by managing minimum and normal indoor temperature setpoints of thermostats, the state of charge in battery energy storage systems (BESS), etc.
Thermal Comfort
Most of the models in the field do not include the thermal comfort topic under their scope. In DREEM, there is a dedicated component responsible for determining appropriate indoor thermal conditions and temperature ranges that result in thermal satisfaction of the occupants based on the “DIN EN ISO 7730”, the “ASHRAE 55”, and the “EN 15251” standards. The model also considers metabolic rates and clothing parameters of occupants.
Impact
The DREEM model has been developed and applied in the context of the following European Commission-funded projects (past and ongoing, Horizon 2020, Horizon Europe, LIFE CET): TRANSrisk, SENTINEL, SENSEI, SocialWatt, ENCLUDE, Tipping+, ENPOR, DigiBUILD, FOrTESIE, ENSMOV+, RENOVERTY, SRI2MARKET, INHERIT.
In addition, the model is often used by the Greek Ministry of Energy and Environment for the revisions of the National Energy and Climate Plan (NECP) and other policy documents.
Publications
- Scientific Articles and Technical Reports
- Stavrakas, V., & Flamos, A. (2020). A modular high-resolution demand-side management model to quantify benefits of demand-flexibility in the residential sector. Energy Conversion and Management, 205, 112339. https://doi.org/10.1016/j.enconman.2019.112339.
- Serafeim Michas, Nikos Kleanthis, Vassilis Stavrakas, Amanda Schibline, Andrzej Ceglarz, Alexandros Flamos, Dimitra Tzani, Dimitris Papantonis, Leonidas Kliafas, Diana Süsser, Johan Lilliestam, Miguel Chang, Jakob Zinck Thellufsen, Henrik Lund, Souran Chatterjee, Gergely Molnar, Diána Ürge-Vorsatz, Bryn Pickering, Raffaele Sgarlato, … Mark Roelfsema. (2022). Report on model application in the case studies: challenges and lessons learnt: Deliverable 7.2. Sustainable Energy Transitions Laboratory (SENTINEL) project (Final). Zenodo. https://doi.org/10.5281/zenodo.7085526.
- Ilias Tsopelas, Vassilis Stavrakas, & Alexandros Flamos. (2022). Model adjustments and modifications to match emerging energy citizenship trends and patterns: Deliverable 5.1. Energy Citizens for Inclusive Decarbonization (ENCLUDE). Zenodo. https://doi.org/10.5281/zenodo.7094196.
- Koasidis, K., Marinakis, V., Nikas, A., Chira, K., Flamos, A., Doukas, H., 2022. Monetising behavioural change as a policy measure to support energy management in the residential sector: A case study in Greece. Energy Policy 161, 112759. https://doi.org/10.1016/J.ENPOL.2021.112759.
- Presentations
- Energy transition in the residential sector in Greece: Investing in natural gas or in electrification?
- Energy transition in the European residential sector: Investigating the saving potential and cost effectiveness of different energy-efficiency measures
- An energy transition dilemma in the Peloponnese region in Greece: Is investing in new natural gas infrastructure the right direction?
- Is just transition in the Coal and Carbon Intensive Region (CCIR) of Megalopolis feasible?
Video Presentation
Relevant Links
GitHub Repository
The DREEM model is open access under the “GNU AFFERO GENERAL PUBLIC LICENSE”. You can find the model here.