The Agent-based Technology adOption Model (ATOM) is an agent-based model, which based on the plausibility of its results compared to historical data and observations, simulates the expected effectiveness of various policy schemes on technology adoption (e.g., small-scale solar PV, battery energy storage systems, heat pumps, electric vehicles, etc.) in the residential sector, for the geographical and socioeconomic context under study. Apart from exploring the expected effectiveness of technology adoption under policy schemes of interest, the model allows us to consider and explicitly quantify the uncertainties that are related to agents’ preferences and decision-making criteria (i.e., behavioural uncertainty). As agents in the model, we refer to consumers/citizens and households.
Key Features
ATOM comprises three (3) primary modelling modules (Figure 1):
- A “calibration” module that establishes the key parameters governing agents’ behaviour and their appropriate value ranges, based on historical data and observations.
- A “sensitivity analysis”module that quantifies uncertainties related to agents’ characteristics and decision-making criteria rather than the more obvious uncertainties based on the calibration results.
- A “scenario analysis” module that examines the potential behaviour of technology adopters in different geographic and socioeconomic contexts, considering different policy schemes of interest through forward-looking simulations.
The novelty of ATOM compared to existing models lies in obtaining realistic uncertainty bounds and splitting the total model’s output uncertainty in its major contributing sources, based on a variance decomposition framework and an uncertainty characterisation method, while accounting for structural uncertainty. Thus, ATOM supports the definition of uncertainty ranges, considering the type (i.e., input, parametric, and structural) and the nature of uncertainty (i.e., epistemic or aleatory), and how uncertainty propagates to the model’s outcomes over the planning time horizon.
Variance decomposition takes place for all the three (3) main modules of ATOM (i.e., calibration, sensitivity analysis, and scenario analysis). By allowing the user to select preliminary values for the agent-related parameters according to the plausibility of its results based on historical data and observations (goodness-of-fit statistics), the model captures input uncertainty (i.e., calibration module). By deriving forward-looking simulations for different behavioural profiles (i.e., different set of agent-related parameters), from willing to invest to risk-averse consumers, ATOM captures parametric uncertainty (i.e., scenario analysis module). Both types of uncertainty are then propagated through the model, and their contribution to the total model’s output variance is quantified. The rest uncertainty is assumed to be explained by the model’s structure. Note that the uncertainty propagation for the agent-related parameters is done for each one of them, allowing calculation of the sensitivity of each parameter to the model’s output, in the context of a variance-based sensitivity analysis (Sobol method), and calculation of the relative contribution of the variance for each parameter to the total model output’s variance (i.e., sensitivity analysis module).
The temporal resolution of the model is typically one (1) month, since technology capacity additions are calculated and presented as such, however it could also be one (1) year, or five (5) years, depending on the time horizon of interest for each application under study.
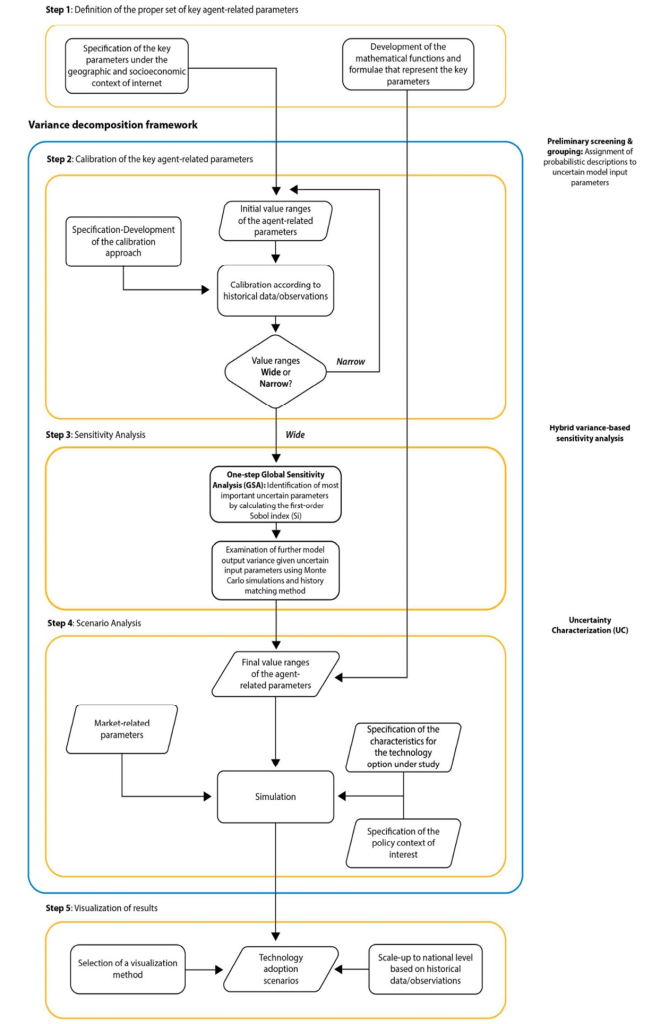
Types of Indicative Applications
Adoption Scenarios for Small-Scale PV and Battery Storage Systems
ATOM has been used for calculating future adoption scenarios for small-scale PV and battery storage systems in the residential sector, modelling households as different interacting agents and their decision-making process in investing in small-scale PV and battery storage systems, and then upscaling at the national level (or local, regional, depending on the spatial resolution of each application at hand), to explore the achievement of capacity targets by 2030.
The model also calculates the CO2 emissions omitted from the decision of consumers/households to invest in mitigation technologies. For example, in the case of small-scale PV systems, yearly projections on PV capacity additions towards 2030 and the achievement of the respective targets in Greece can be calculated using ATOM, and emissions omitted due to these new PV capacity additions, using emission factors, are also calculated on a yearly base.
It can also get parameterised based on specifications of exogenous scenario data, e.g., in the case of Greece, scenario specifications from the most recent versions of the National Energy and Climate Plan and the Long-term strategy 2050 documents are used.
In addition, socioeconomic narratives could also affect the parameterisation of ATOM directly, but mostly indirectly through assumptions, based on which parameterisation happens. For example, a “power to the people” socioeconomic narrative could be translated to more decentralised structures of energy consumption, e.g., energy communities, energy hubs, etc. This means that households will invest in small-scale solar PV and potentially storage systems, or that will be willing to participate in novel regulatory frameworks that valorise self-consumption so that they become more energy autonomous.
Impact
The ATOM model has been developed and applied in the context of the following European Commission-funded projects (past and ongoing, Horizon 2020, Horizon Europe, LIFE CET): TRANSrisk, SENTINEL, ENCLUDE.
Publications
- Scientific Articles and Technical Reports
- Stavrakas, V., Papadelis, S., & Flamos, A. (2019). An agent-based model to simulate technology adoption quantifying behavioural uncertainty of consumers. Applied Energy, 255 (22). https://doi.org/10.1016/j.apenergy.2019.113795.
- Michas, S., Stavrakas, V., Papadelis, S., & Flamos, A. (2020). A transdisciplinary modelling framework for the participatory design of dynamic adaptive policy pathways. Energy Policy, 139 (4). https://doi.org/10.1016/j.enpol.2020.111350.
- Michas, S., Kleanthis, N., Stavrakas, V., Schibline, A., Ceglarz, A., Flamos, A., et. al. (2022). Model application in the case studies: challenges and lessons learnt. Deliverable 7.2. Sustainable Energy Transitions Laboratory (SENTINEL) project. University of Piraeus Research Centre (UPRC), Piraeus, Greece. https://zenodo.org/records/7085526.
- Ilias Tsopelas, Vassilis Stavrakas, & Alexandros Flamos. (2022). Model adjustments and modifications to match emerging energy citizenship trends and patterns: Deliverable 5.1. Energy Citizens for Inclusive Decarbonization (ENCLUDE). Zenodo. https://doi.org/10.5281/zenodo.7094196.
- Presentations
Relevant Links
GitHub Repository
ATOM is open access under the “GNU AFFERO GENERAL PUBLIC LICENSE”. You can find the model here.