Classic decision-making used to be based on a static plan that was considered optimal for the “most likely” future outcome. This approach has proven to be vulnerable to unexpected future evolutions, which often led to failure of plans that were considered optimal. With adaptive policies and strategies, the focus is on short-term planning, with simultaneous description of potential future adaptive actions that can be deployed in the event of unlikely contextual evolutions.
The Adaptive polIcymaking Model (AIM) is a decision support model which enables the exploratory analysis of policy/strategy pathways towards the achievement of one or multiple targets, identifying in parallel their conditions of success. To do so, AIM performs meta-analysis of simulations models’ outputs, and evaluates the performance of selected policies/strategies over many combinations of a large number of contextual uncontrollable variables (scenarios). Then, it visualizes successful policy pathways towards a predefined target, and sets up a monitoring system for real-world policy adaptations in case of unexpected contextual future evolutions.
Key Features
The novelty of AIM lies in (i) using a simple clustering logic, thus it can be easily adapted for soft-linking with a wide variety of models, (ii) generating adaptive policies for different contexts, by changing the limits of the uncontrollable variables (scenarios), making it a useful tool for application at various scales and contexts, and (iii) facilitating interactive stakeholder consultation for the design of policy pathways, through real-time and easily interpretable visualizations.
Overall AIM:
- Performs exploratory analysis of policy/strategy options, to identify those that perform well under deep uncertainty, while specifying coping strategies that can be implemented in the case of realization of unlikely uncertainties.
- Supports adaptive policy making, by specifying why and when a policy change should be sought, which policies/strategies are prone to specific uncertainties, and therefore making explicit what should be monitored to trigger adaptation during actual implementation.
- Supports, stepwise implementation of policies. This means that a policy or strategy may be chosen for implementation for a specific period of time by a stakeholder, and the results, as well as the plausible policy/strategy pathways forward, are updated almost instantly. With this feature, a tight participatory modelling process is feasible.
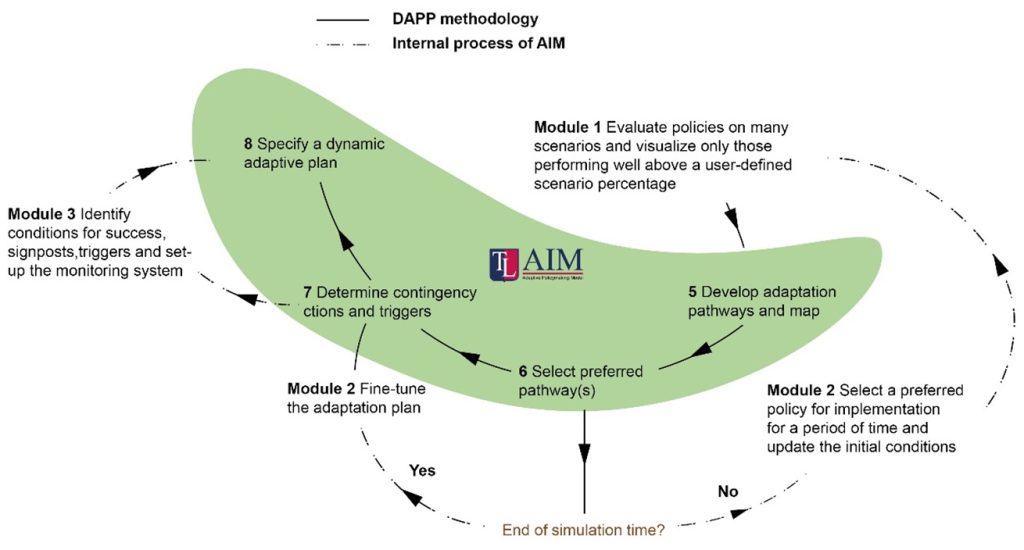
All the modules of AIM are developed using the Python language.
Why AIM?
AIM, enables the fast assessment of a large number of energy transition pathways along an analysis horizon, without mandating the same number of simulations to be performed by computational- and time-intensive simulation models. Specifically, it enables the assessment of pm policy/strategy pathways with only p∙m simulations performed by a simulation model, where p is the number of policies under investigation and m the number of years in the analysis horizon. Therefore, even if a simulation model requires a significant amount of time to simulate a year of analysis, AIM can be used to augment the analysis space.
Main Functionalities
Simultaneous assessment of multiple policy outcomes: AIM features an interactive interface which allows for inter-sectoral or cross-sectoral analyses. This allows multiple policy outcomes to be presented in the interactive interface and comparatively assessed simultaneously.
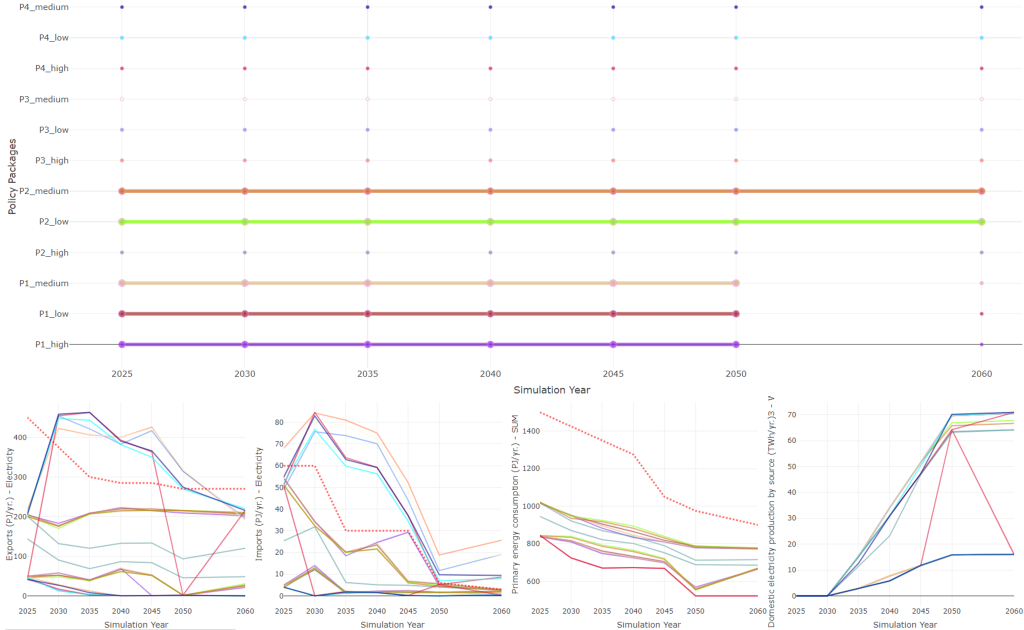
Clustering with respect to multiple targets: AIM identifies the value range of contextual factors, under the effect of which the assessed policies/strategies simultaneously satisfy all targeted outcomes. Furthermore, the decomposition of target clustering is also possible. This means that the contextual space under the effect of which a policy performs well with respect to each target individually is distinguished. This functionality provides insight into the target-specific ‘sensitivity’ of policies to context evolutions.
Multiple policy implementation and assessment: In real-world situations, it is common for more than one policy to be implemented simultaneously. AIM supports policy package implementations. After implementing a policy for a specific time period, users can implement another one, starting concurrently or later than the previously implemented policy, and extending until any timeframe within the assessment period.
Impact
The AIM model has been developed and applied in the context of the following projects funded by the European Commission and the Swiss Federal Office for Energy: TRANSRisk, SENTINEL, TIPPING+, POLIZERO.
Scientific Articles and Other Relevant Publications
- Michas, S., Stavrakas, V., Papadelis, S., & Flamos, A. (2020). A transdisciplinary modeling framework for the participatory design of dynamic adaptive policy pathways. Energy Policy, 139, 111350. https://doi.org/10.1016/j.enpol.2020.111350
- Michas, S., & Flamos, A. (2023). Are there preferable capacity combinations of renewables and storage? Exploratory quantifications along various technology deployment pathways. Energy Policy, 174, 113455. https://doi.org/10.1016/j.enpol.2023.113455
Video Presentation
A study performed with AIM, soft-linked with the STREEM and BSAM models, is presented in the video below.